
The answers above point out how the block size can impact performance and suggest a common heuristic for its choice based on occupancy maximization.
Cuda dim3 3dimension compute 5.0 code#
The good news is that because you are working in multiples of the warp size, the search space is very finite and the best configuration for a given piece of code relatively easy to find. By benchmarking, you will probably find that most non-trivial code has a "sweet spot" in the 128-512 threads per block range, but it will require some analysis on your part to find where that is. There are people writing PhD theses around the quantitative analysis of aspects of the problem (see this presentation by Vasily Volkov from UC Berkley and this paper by Henry Wong from the University of Toronto for examples of how complex the question really is).Īt the entry level, you should mostly be aware that the block size you choose (within the range of legal block sizes defined by the constraints above) can and does have a impact on how fast your code will run, but it depends on the hardware you have and the code you are running. The second point is a huge topic which I doubt anyone is going to try and cover it in a single StackOverflow answer. The orthodox approach here is to try achieving optimal hardware occupancy (what Roger Dahl's answer is referring to). Each streaming multiprocessor unit on the GPU must have enough active warps to sufficiently hide all of the different memory and instruction pipeline latency of the architecture and achieve maximum throughput.The number of threads per block should be a round multiple of the warp size, which is 32 on all current hardware.How each code behaves will be different and the only real way to quantify it is by careful benchmarking and profiling. The number of threads per block you choose within the hardware constraints outlined above can and does effect the performance of code running on the hardware. If you stay within those limits, any kernel you can successfully compile will launch without error. Each block cannot consume more than 16kb/48kb/96kb of shared memory (Compute.The maximum dimensions of each block are limited to.Each block cannot have more than 512/1024 threads in total ( Compute Capability 1.x or 2.x and later respectively).If you exceed any of these, your kernel will never run. Appendix F of the current CUDA programming guide lists a number of hard limits which limit how many threads per block a kernel launch can have. One part is easy to quantify, the other is more empirical.
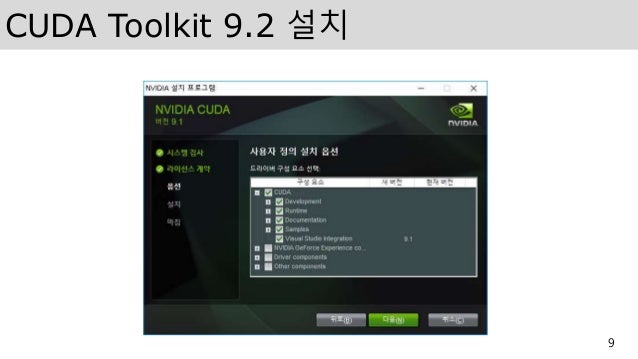
There are two parts to that answer (I wrote it).
